Leveraging Predictive Analytics for Better Business Decisions
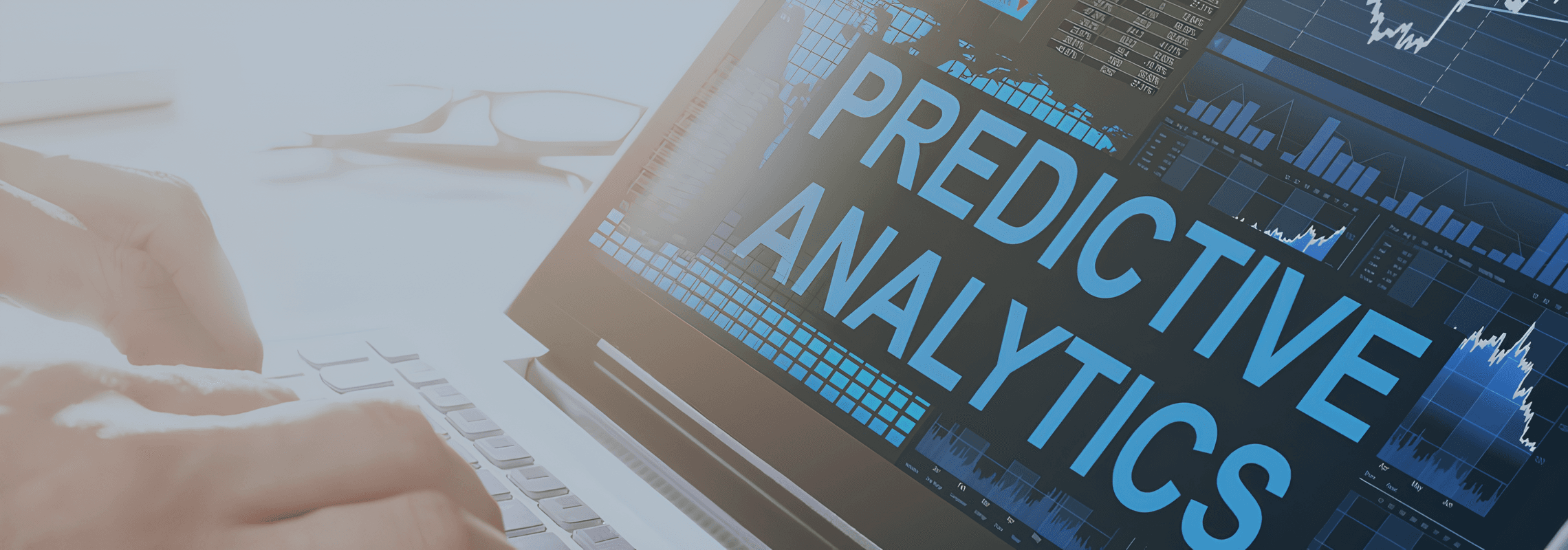
In today’s data-driven world, businesses increasingly use predictive analytics to gain a competitive edge. By leveraging vast data, predictive analytics allows organizations to make informed decisions, optimize operations, and improve customer experiences. This article explores the benefits and applications of predictive analytics across various industries, highlighting how it can transform business intelligence and decision-making processes.
What is Predictive Analytics?
Predictive analytics involves using statistical techniques, machine learning algorithms, and data mining to analyze historical data and make predictions about future events. It aims to uncover patterns and relationships within data sets to forecast trends, behaviors, and outcomes. This process often involves several steps, including data collection, data preprocessing, model selection, and validation.
Benefits of Predictive Analytics
1. Improved Decision-Making: Predictive analytics provides businesses with insights that support strategic decision-making. By understanding potential future scenarios, companies can plan better and mitigate risks.
2. Cost Reduction: By predicting maintenance needs, supply chain disruptions, and customer behavior, businesses can reduce operational costs and avoid unnecessary expenditures.
3. Enhanced Customer Experience: Predictive models can help identify customer preferences and behaviors, allowing businesses to tailor their offerings and improve customer satisfaction.
4. Competitive Advantage: Organizations that leverage predictive analytics can stay ahead of their competitors by anticipating market trends and responding proactively.
5. Risk Management: Predictive analytics helps in identifying and mitigating potential risks, whether they relate to financial markets, customer creditworthiness, or operational failures.
Applications of Predictive Analytics
1. Finance
In the financial sector, predictive analytics is used extensively for risk management, fraud detection, and investment strategies. Financial institutions analyze historical transaction data to predict credit risks and detect fraudulent activities in real time. For example, banks use predictive models to determine the likelihood of loan defaults and to design personalized investment portfolios for clients.
2. Healthcare
Predictive analytics is revolutionizing healthcare by improving patient outcomes and operational efficiency. Healthcare providers use predictive models to forecast disease outbreaks, personalize treatment plans, and manage hospital resources effectively. For instance, predictive analytics can identify patients at high risk of developing chronic conditions, allowing for early interventions and better care management.
3. Retail
Retailers leverage predictive analytics to optimize inventory management, enhance customer engagement, and increase sales. By analyzing purchase history and customer preferences, retailers can predict future buying patterns and personalize marketing campaigns. Predictive models also help in managing supply chains by forecasting demand and reducing stockouts.
4. Manufacturing
In manufacturing, predictive analytics is used for predictive maintenance, quality control, and supply chain optimization. By analyzing equipment data, manufacturers can predict failures and schedule maintenance proactively, reducing downtime and operational costs. Predictive models also help in ensuring product quality by identifying potential defects early in the production process.
5. Telecommunications
Telecommunications companies use predictive analytics to improve customer retention, optimize network performance, and enhance service delivery. By analyzing usage patterns and customer feedback, telecom providers can predict churn and implement strategies to retain customers. Predictive models also help in optimizing network resources and ensuring high-quality service delivery.
6. Energy
The energy sector uses predictive analytics to forecast energy demand, optimize grid operations, and manage resources efficiently. Predictive models help in balancing supply and demand, reducing energy wastage, and planning infrastructure investments. For example, utilities can predict peak usage times and adjust their operations to meet demand without overloading the grid.
7. Marketing
Marketers use predictive analytics to segment audiences, personalize campaigns, and improve ROI. By analyzing customer behavior and engagement data, marketers can predict the success of campaigns and allocate resources more effectively. Predictive models help in identifying high-value customers and designing targeted marketing strategies.
8. Human Resources
Predictive analytics is transforming HR by improving talent acquisition, employee retention, and workforce planning. By analyzing employee data, HR professionals can predict turnover, identify high performers, and develop strategies to retain talent. Predictive models also help in planning training programs and succession planning.
9. Transportation and Logistics
Predictive analytics plays a crucial role in optimizing transportation and logistics operations. By analyzing traffic patterns, weather data, and shipment history, logistics companies can predict delays and optimize routes. Predictive models help in reducing transportation costs, improving delivery times, and enhancing customer satisfaction.
10. Insurance
In the insurance industry, predictive analytics is used for risk assessment, pricing strategies, and fraud detection. Insurers analyze historical claims data to predict the likelihood of future claims and set premiums accordingly. Predictive models also help in identifying fraudulent claims and mitigating financial losses.
Key Technologies and Tools in Predictive Analytics
1. Machine Learning Algorithms: Algorithms such as regression analysis, decision trees, and neural networks are commonly used in predictive analytics to identify patterns and make predictions.
2. Data Mining: Data mining techniques involve exploring large datasets to uncover hidden patterns and relationships that can be used for predictive modeling.
3. Statistical Analysis: Statistical methods are used to validate predictive models and ensure their accuracy and reliability.
4. Big Data Technologies: Technologies like Hadoop and Spark enable the processing of massive datasets, making it possible to analyze large volumes of data for predictive analytics.
5. Business Intelligence Platforms: BI tools such as Tableau, Power BI, and QlikSense help in visualizing predictive analytics results and making data-driven decisions.
Challenges in Predictive Analytics
1. Data Quality: The accuracy of predictive models depends on the quality of data. Incomplete or inaccurate data can lead to incorrect predictions.
2. Complexity: Developing and implementing predictive models can be complex and requires expertise in data science and machine learning.
3. Privacy Concerns: The use of personal data for predictive analytics raises privacy concerns and requires adherence to data protection regulations.
4. Integration: Integrating predictive analytics into existing business processes and systems can be challenging and requires careful planning.
Future Trends in Predictive Analytics
1. AI and Machine Learning: The integration of AI and machine learning will continue to enhance the capabilities of predictive analytics, making it more accurate and efficient.
2. Real-Time Analytics: The demand for real-time predictive analytics will grow, enabling businesses to make immediate decisions based on current data.
3. Cloud Computing: Cloud-based predictive analytics solutions will become more popular, offering scalability and flexibility.
4. Automated Predictive Analytics: Automation will simplify the development and deployment of predictive models, making predictive analytics accessible to a broader range of users.
5. IoT Integration: The integration of IoT data with predictive analytics will provide new opportunities for real-time monitoring and decision-making.
Conclusion
Predictive analytics is a powerful tool that can transform business intelligence and decision-making processes across various industries. By leveraging predictive models, businesses can gain valuable insights, optimize operations, and stay ahead of the competition. As technology continues to evolve, the applications and benefits of predictive analytics will only expand, making it an essential component of modern business strategy.
For more information on predictive analytics and how it can benefit your business, explore resources from the industry and book a demo on the main page of Edsson site.
Featured content
How DevOps is Revolutionizing Software Development
Denys Seletskyi
Digital Transformation in Retail: Key Trends for 2024
Denys Seletskyi
The Future of AI in Financial Services
Denys Seletskyi
Edsson's AI-Assisted Software Development: A New Paradigm for Accelerating Time-to-Market
Denys Seletskyi
Denys Seletskyi
Marketing Specialist with 7+ years of experience in digital-marketing researcher, strategist and execute. Have huge working experience with big brands in multicultural teams by powerful frameworks, like SOSTAC or RACE, in SCRUM Marketing Team, which increases the efficiency of the marketing department almost in every company thank to focuses workflow in 1-week sprint.